The following article was written by Dr. Cornelia C. Walther, a visiting scholar at Wharton and director of global alliance POZE. A humanitarian practitioner who spent over 20 years at the United Nations, Walther’s current research focuses on leveraging AI for social good.
On April 8, 2025, a driverless Zoox robotaxi misjudged an approaching vehicle, braked too late, and sideswiped it at 43 miles per hour on the Las Vegas Strip. One month later, the Amazon subsidiary issued a software recall on 270 autonomous vehicles and suspended operations while regulators investigated the code. Though the crash was minor, it sent a strong message: When AI systems fail, responsibility quickly shifts from programmers to corporate leadership to regulators.
This incident highlights a growing challenge for business leaders. While four out of five finance chiefs consider AI “mission-critical,” the vast majority admit they still struggle to monetize it, according to a recent DigitalRoute survey. Beyond revenue concerns lies a significant governance gap — 71% of technology leaders don’t trust their organizations to manage future AI risks effectively, based on Kyndryl’s 2025 AI Readiness Report. But this does not prevent them from integrating it into their operations. Is there a disconnect between the aspiration to do the right thing and doing things the right way?
The M4-Matrix: A Framework for AI Accountability
Accountability cannot be an afterthought, activated only in the wake of a crisis. It must be embedded from the outset and upheld at every stage — from design to deployment and oversight. This article explores the accountability challenge posed by the rapid rise of AI, using the four-level M4-Matrix to offer a structured framework. Examining the micro (individual), meso (organizational), macro (governmental), and meta (global) levels provides an integrated perspective for assessing interdependencies and assigning responsibility across all layers of action.
Level | Key Question | Typical Actors |
Micro | Did individuals design, deploy, or use the tool responsibly? | Frontline users, data scientists, software engineers, and users |
Meso | Did the organization provide proper guardrails and incentives? | Corporations, hospitals, banks, and startups |
Macro | Do national laws align profits with protections? | Legislators, regulators, and courts |
Meta | Are global norms preventing a regulatory race to the bottom? | UN agencies, OECD, and multi-stakeholder alliances |
Accountability is a supply chain, not a patchwork. Each level must operate in sync with the others. If they do, it’s a win-win-win-win for each component and the whole they constitute. Below, we look at each level and the features that are involved in making them a sound piece of the accountability stream.
The Micro Level: Humans in the Loop
The micro level of accountability focuses on individual responsibility in building and using AI systems. Take the case of Northwell Health’s radiology department, which implemented an AI-assisted diagnostic tool in early 2025. While the system demonstrated 93% accuracy in detecting early-stage lung nodules in clinical trials, its real-world performance varied dramatically across the hospital network’s 23 facilities. Investigation revealed that radiologists at high-performing locations received specialized training on interpreting the AI’s confidence scores and understood when to question the system’s findings. Meanwhile, at facilities with poorer outcomes, practitioners either overrelied on the AI (accepting its conclusions without scrutiny) or largely ignored its inputs (defaulting to traditional methods regardless of the AI’s insights). The technology was identical, but the human-AI interaction determined success or failure. AI without NI (natural intelligence) is like building a Porsche and giving it to people without a driver’s license; to thrive in an AI-infused context requires HI (hybrid intelligence).
Effective safeguards at the micro level include:
- Double literacy, or investing in both human literacy — a holistic understanding of self and society — and algorithmic literacy.
- Transparent documentation that involves creating comprehensive logs that capture data exclusions, algorithm adjustments, and edge-case rationale, not black boxes.
- Measured human oversight to record when, why, and by whom safeguards are bypassed.
- Incentivized vigilance that links bonuses to safe-use metrics, not just deployment speed.
The Meso Level: Corporate Duty of Care
Three years after the launch of ChatGPT, the era of AI experimentation is over. We have entered the stage of systematic integration, which should be accompanied by streamlined accountability for the outcomes, internally and at scale. This is not happening yet. An analysis of nearly 75,000 Hugging Face repositories found that less than one in two included model card documentation, which provides important information about AI models and is a fundamental element for downstream safety.
Organizations demonstrating best practices typically implement:
- Intersectional collaboration that harnesses the expertise of teams and dissolves siloes.
- Pre-mortems that stress-test vulnerabilities like prompt injection, data drift, and adversarial examples before launch.
- Living documentation that updates model cards whenever systems are retrained or repurposed.
- Regular simulation exercises that mirror cybersecurity tabletop drills to practice detection, rollback, and disclosure procedures.
Ethical concerns and institutional responsibility can neither be delegated to an LLM nor can they be outsourced. Using AI internally implies standing up for its outcomes externally.
The Macro Level: Regulatory Framework
2025 has proven to be a watershed year for AI regulation. The EU AI Act’s first substantial obligations took effect on February 2, 2025, for high-risk systems, while U.S. regulators rely on industry-specific laws and the NIST AI Risk Management Framework, whose February 2025 revision (IR 8011r1) added testable controls for continuous monitoring.
In the past, successful regulatory approaches tended to:
- Reflect the values and concerns of citizens as expressed by voters, collected via memoranda or tracked via social media.
- Require substantive evidence through test datasets, robustness scores, and data-provenance chains.
- Scale enforcement based on market power and risk level — for example, health care AI faces stricter scrutiny than entertainment applications.
- Provide regulatory sandboxes so startups can innovate under supervision rather than in regulatory uncertainty.
However, regulators today are navigating uncharted territory, and do not have the luxury to wait for conclusive evidence of risks and an expansive layout of their underlying causes and consequences.
The Meta Level: Building Global Norms
International frameworks are set to gain momentum in 2025 with the UNESCO Global Forum on the Ethics of AI, which will gather 60 countries to benchmark national Readiness Assessments, and the G20 Digital Ministers’ Roadmap for AI Governance, which builds on the OECD AI Principles and is expected in December 2025.
While these instruments remain voluntary, they exert influence through procurement requirements and trade agreements that incorporate standards like audit logs and security testing into market access conditions. They are also setting an ethical standard for companies and consumers.
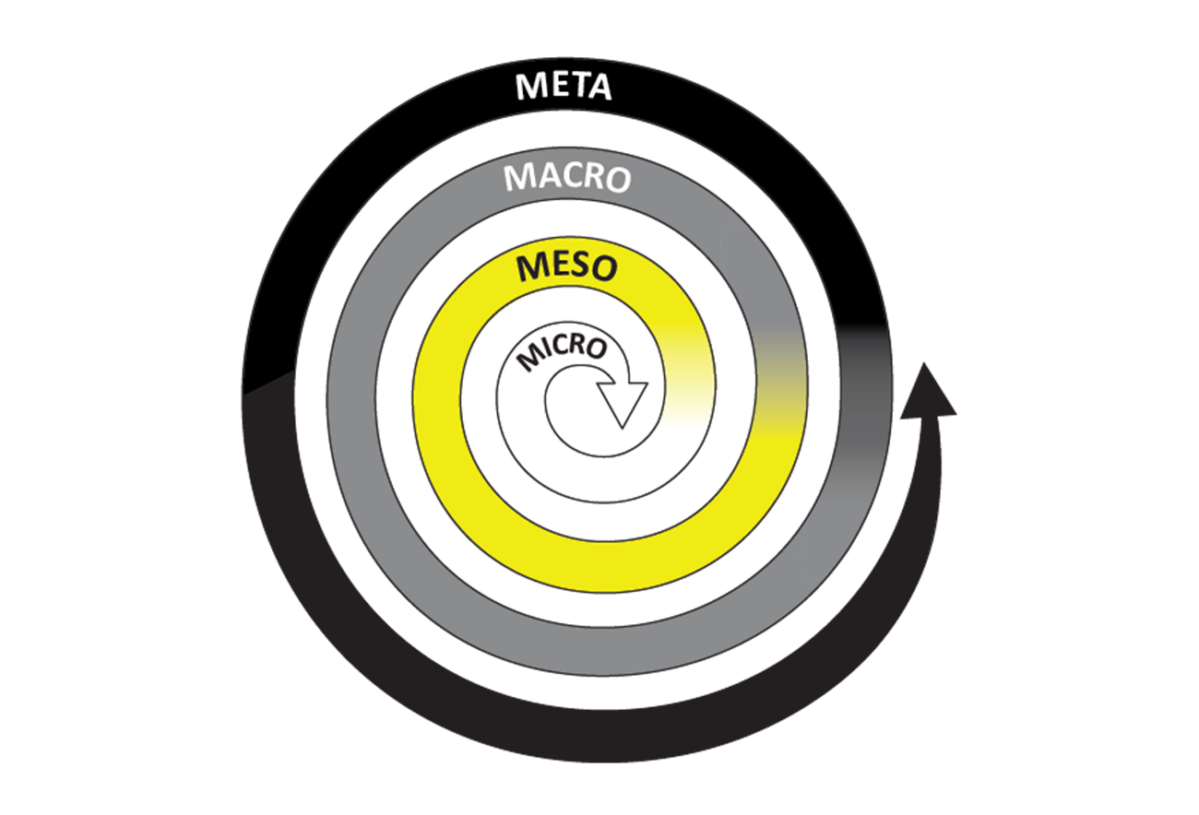
What Can Business Leaders Do Today?
Executive confidence in AI remains high despite limited immediate revenue gains, reflecting a deeper understanding of the values required to unlock its full potential. A 2025 McKinsey workplace survey found that while only 19% of C-suite leaders have achieved revenue increases over 5% from AI to date, a significant 92% intend to increase investment. This commitment suggests a recognition that the true, enduring value of AI development depends critically on the mindsets and foundational values that underpin its creation and application.
By consciously prioritizing prosocial values in AI development, companies have a genuine opportunity to move beyond purely financial metrics and actively shape positive outcomes, establishing themselves as a force for good. This necessitates deliberate leadership. Maybe the question is not so much what value we are optimizing for, but which values we are choosing in the first place, and what aspirations guide that choice.
The A-Frame for Accountable AI
Accountability in the age of AI is not a fixed destination — it’s a dynamic process shaped by complex interdependencies, many of which remain invisible until a system fails. But leaders don’t have to wait for a crisis to act. There are practical steps that can be taken today, across industries and levels of leadership. One starting point is the A-Frame — a simple yet powerful lens that grounds AI responsibility in four key actions.
A | Leadership Action |
Awareness | Map both capabilities and failure modes — technical, social, and legal. You can’t manage what you don’t fully understand. |
Appreciation | Recognize that accountability flows across the entire M4-Matrix (micro, meso, macro, meta). Retroactive finger-pointing is a symptom of failed foresight. |
Acceptance | Acknowledge that zero-failure systems are a myth. Instead, design for resilience, with graceful degradation and recovery paths. |
Accountability | Define ownership clearly. Assign responsible roles, measurable KPIs, and transparent escalation protocols — before launch, not after. |
There’s one more “A” that future-ready leaders should not overlook: Aspiration. AI isn’t just a tool for automation — it’s a lever for transformation. When deliberately designed and deployed to enhance not only efficiency but also ethics, AI becomes a force multiplier for human potential. Businesses that align their AI strategies with broader human values stand to gain not just a competitive advantage but lasting legitimacy. In a rapidly evolving field, aspirations anchored in purpose — not just profit — offer the most reliable compass.
Accountability as a Network Effect
The complexity of AI systems doesn’t dilute human responsibility — it expands the need for precision and foresight. The recent Zoox recall is a case in point: A minor malfunction in an autonomous system triggered widespread scrutiny, underscoring how small failures can become systemic headlines. In an interconnected world, accountability scales like a network effect.
Organizations that can trace the arc of responsibility — from code commits to boardroom decisions, from technical implementation to global governance — will be more resilient, more trusted, and less vulnerable to legal or reputational fallout.
Distributed technical work does not mean distributed ethical responsibility. It heightens the urgency to define and own it. Accountability in AI is not automatic. It’s a choice. But it’s a choice we must make, together and now.