The following article was written by Scott A. Snyder, a senior fellow at Wharton, adjunct professor at Penn Engineering, and chief digital officer at EVERSANA; and Hamilton Mann, group vice president, digital marketing and digital transformation at Thales.
With the weekly drumbeat of generative AI advancements and corporate leaders signaling the need for their organizations to harness the power of AI, larger questions are emerging for these same executives to address.
In addition to the ethical challenges that AI presents for their customers, employees, and society, companies must grapple with how AI will fundamentally shift their operating model, including the workforce. Almost two-thirds (65%) of American executives believe generative AI will have a high or extremely high impact on their organization in the next three to five years, but 60% say they are still one to two years from deploying their first GenAI solution, per a recent KPMG survey.
Ignoring the seismic shift brought about by AI — particularly with Large Language Models (LLMs) like ChatGPT — is no longer a viable option for organizations. The torrential rise of AI, championed by industry titans such as OpenAI, Google, Meta, Microsoft, and Nvidia, is rapidly reshaping how work gets done and how companies operate and deliver value to their customers and shareholders.
Let’s explore the key components of operating models that are most profoundly transformed by AI’s influence: organization, people, processes, technology, and culture.
Organization: Blueprint Over Structure
In an era dominated by rapid AI advancements, it’s crucial to assess the impact on the entire organizational blueprint, rather than merely the organizational structure. The forward-looking and comprehensive nature of a blueprint, designed for adaptability, offers a more inclusive approach that anticipates future changes and can seamlessly integrate AI’s transformative potential into the very fabric of an organization’s operations and strategy.
Preparing an organization for AI is less a matter of stringent modification and more about fluidity. Fluid organizations are capable of tapping into central expertise and data assets to augment AI skills and innovate. As opposed to brittle, hierarchical organizational structures, fluid organizations follow the 80/20 rule, able to respond to 80% of predictable events while remaining flexible enough to navigate the 20% of unforeseen challenges.
The primary challenge lies in establishing a dynamic organizational structure that optimizes efficiency in addressing these regular tasks, allowing AI to enhance productivity while maximizing agility in responding to opportunities and blind spots.
Culture: Mindset Over Skillset
When companies embarked on their digital transformation journeys, they saw the need to double down on digital literacy and deep technology to achieve their objectives. But this era of AI requires a different emphasis — one that focuses on mindset over skillset. Rather than cultivating an army of data scientists and programmers, companies need to evolve their culture to embrace continual experimentation, responsible innovation, and the potential of AI to drive lasting impact for employees, customers, and communities.
Moreover, it’s imperative to acknowledge that AI is more than just a technology or tool. It has the capacity to learn, adapt, and even make decisions based on the data and content it has access to, creating a unique superpower for end-users that requires both creativity and caution in how it’s deployed. Blind reliance on AI without a clear sense of purpose or ethical considerations is sure to increase the risk of doing harm to the business and the people it serves.
True leadership in an AI-first future isn’t about tech prowess but the ability to integrate technology meaningfully into the broader objectives of the organization, ensuring it aligns with human values and positive societal impact. Leaders must instill a mindset that allows the organization to push itself and challenge the status quo. AI must be approached not as an infallible oracle but as a powerful ally that, when used with discernment, can amplify human capacities.
Lastly, it’s essential to understand that the very essence of any digital technology is its evolutionary nature. What may be a groundbreaking innovation today could become obsolete tomorrow. Relying solely on the technical know-how of the present might lead into the trap of shortsightedness. This makes it critical for the culture to be built on continuous learning and adaptability.
“Fluid organizations are capable of tapping into central expertise and data assets to augment AI skills and innovate.”
Process: Data Over Procedure
As AI begins to take on an increasingly dominant role in decision-making, a critical challenge has emerged: understanding the labyrinth of data-driven processes that can be transformed with AI while maintaining trust with end-users.
Leaders must come to terms with the uncomfortable truth that AI’s decision-making capabilities often far exceed human comprehension. Embracing practices like highlighting relevant data that contribute to AI outputs or building models that are more interpretable could enhance AI transparency. Using AI to diagnose a patient in a medical setting with oversight from the clinical staff is an example of how this transparency will be critical.
AI’s effectiveness is heavily influenced by the data it processes. There will inevitably be biases because data are originally produced by humans, and the process of refining them involves humans again. To counter these biases, it’s important to involve diverse teams in data collection and processing while also allowing AI models to learn and adapt from the data, including biases, to respond more genuinely to different perspectives. Rather than trying to irradicate bias, companies need to evolve their processes to acknowledge and manage it, reducing associated risks while advancing their AI innovation efforts.
There is also a trade-off around the size of data sets versus the accuracy and reliability of AI models. While big tech players race towards larger foundational models with over a trillion parameters trained on massive data sets, companies have an opportunity to leverage their own proprietary data sets to develop small, more focused LLMs with higher reliability and accuracy in domains such as content generation or customer assistance. This must be balanced with the recognition that smaller data sets are less likely to encompass broader knowledge and depth of human perspectives.
People: Human Capital Transitioning Over Reskilling
Leaders need to face the new human capital challenge AI poses. While 62% of leaders are optimistic about AI, only 42% of frontline employees share that view, and only 14% have received AI training to date, according to a recent BCG survey. The emergence of AI necessitates new skill sets and competencies, redefining what expertise is essential for delivering value in this new “AI-conomic” era. But it goes beyond that.
The prospect of AI triggering mass unemployment is often overshadowed by optimistic predictions based on past technological revolutions. It is imperative, however, to examine AI’s impact not through the lens of the past, but in the context of its own unique capabilities. The transition from horse-and-buggy to automobiles indeed reshaped job markets, but it did not render human skills redundant. AI, on the other hand, has the potential to do just that.
Contrary to the belief that AI should not create meaningful work products without human oversight, the use of AI in tasks like document or code generation can result in increased efficiency. Of course, human oversight is important to ensure quality, but relegating AI to merely auxiliary roles might prevent us from fully realizing its potential.
Take Collective[i]’s AI system for instance. Yes, it may free salespeople to focus on relationship building and actual selling, but it could also lead to a reduced need for personnel as AI handles an increasingly larger share of sales tasks. The efficiencies of AI could easily shift from job enhancement to job replacement, creating a precarious future for many roles. Similarly, while OpenAI’s Codex may make programming more efficient, it could undermine the value of human programmers in the long run.
Certainly, investments in education and upskilling form a key part of any strategy to cope with job displacement due to AI. This includes fostering digital skills that enable workers to adapt to the changing employment landscape and thrive in AI-dominated sectors. It is also imperative to craft comprehensive social and economic policies that provide immediate and long-term support to those displaced by AI’s advancement. Social support services and career counseling should be made widely available to help individuals navigate through the transition.
Finally, a human capital value transitioning plan can cushion the impact of AI-induced displacement and build a resilient and inclusive organization while safeguarding its human capital.
“Leaders must come to terms with the uncomfortable truth that AI’s decision-making capabilities often far exceed human comprehension.”
Technology: Ethical Stands Over Value Proposition
AI introduces novel policies and standards, necessitating a reevaluation of decision-making protocols and organizational conduct. While having a flexible technology stack and data ecosystem are critical elements for scaling AI innovations, putting in place appropriate ground rules for responsible and ethical AI development is even more critical to ensure companies maximize benefit while minimizing harm to stakeholders.
But the agile nature of AI evolution has outpaced the regulation meant to keep it in check. The burden of ensuring that AI tools are used ethically and safely thus rests heavily on the shoulders of the companies employing them. It is essential for leaders to foster a culture of ethical AI development and usage, and not just depend on external watchdogs or regulation.
It’s not just about reaping the benefits of AI, but also about responsibly integrating these technologies without causing harm to stakeholders. This necessitates not only technological sophistication, but also ethical mindfulness and societal understanding. Zoom, the popular videoconferencing software, illustrates this. The company made headlines and raised concerns when it updated its terms of service to gather customer data to train its artificial intelligence.
The path to responsible AI deployment is less about picking the perfect technology solution and more about creating a technology environment that enables rapid experimentation and ethical use of the technology across the organization. Publishing your own ethical and responsible AI development framework that reflects the core values of your company and puts end-user needs first is a key step towards this path.
Pioneering AI-Driven Operating Models
Organizations will need to chart their own AI-transformation journey by prioritizing an adaptive blueprint over structure, emphasizing mindset more than just skillset, valuing data above procedure, placing emphasis on the transition of human capital value instead of just reskilling, and elevating ethical stances above traditional value propositions as shown in the figure below.
AI is like no other tech wave in history with the potential to empower employees, reimagine work, and shift how companies deliver value in leaps versus incremental steps. Companies that can quickly evolve towards improving their AI Quotient will begin to separate themselves from the pack in their respective industries in terms of speed and impact of AI-driven innovations. But this will require bold leadership and a radical approach to transforming the operating model to unlock AI’s full potential. How ready is your company for the AI-wave? Don’t look now, but it’s already here.
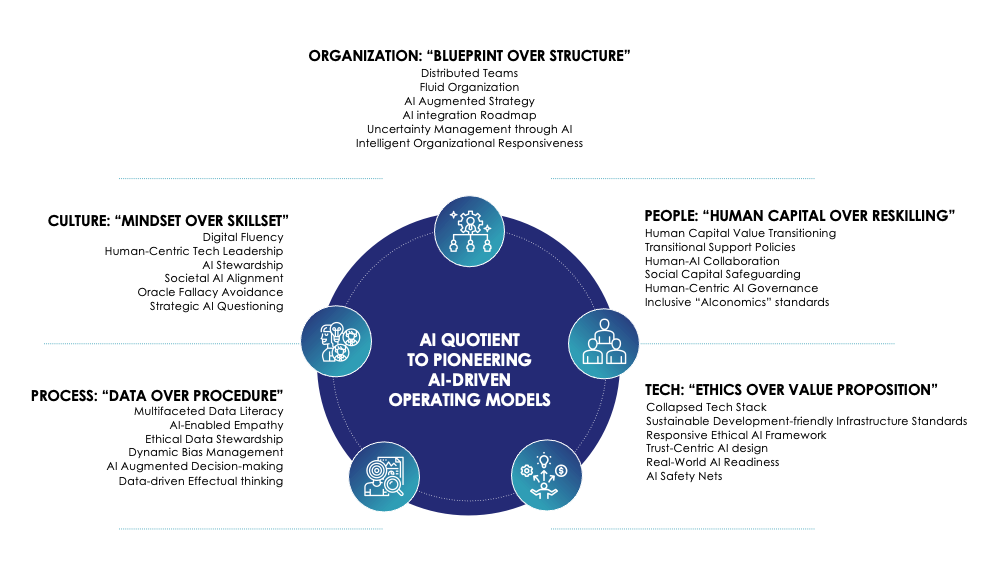