The following article was written by Scott A. Snyder, a senior fellow at Wharton, adjunct professor at Penn Engineering, and chief digital officer at EVERSANA; and Jason Hreha, the founder and CEO of Persona and former Global Head of Behavioral Science at Walmart.
We have all seen the recent reports showing the disconnect between leader expectations and meaningful AI transformation in companies. According to a recent Accenture survey, 98% of business leaders want to adopt AI, but only 10% of companies have gen AI models in production and even less can point to specific AI benefits in their P&L. We have officially moved from the “experimentation” phase to the “rationalization” phase with companies looking for use cases with real business impact. Easier said than done. Measuring the true return on AI investments (ROAI) has been harder than expected as projecting the actual life cycle costs for standing up AI solutions is a bit of a moving target, and measuring true benefits for AI solutions — whether its hours saved or improved job satisfaction — has proved to be just as challenging. And even when we are able to measure the ROAI, companies are not seeing anywhere near the theoretical gains projected by some of the top consulting firms, industry analysts, and academic experts.
To understand why these organizational efforts often stall, we need to examine the day-to-day behaviors and perceptions that ultimately determine whether AI is fully adopted — or quietly sidelined.
The AI Knowing-doing Gap
According to a recent study by Slack on the impact of AI on the workforce, 76% of workers felt the urgency to become AI experts, but only 33% of workers are adopting AI in their everyday activities. More surprising is that nearly half (48%) of all desk workers would be uncomfortable admitting to their manager that they used AI for common workplace tasks, with the top reasons cited being: 1) feeling like using AI is cheating, 2) fear of being seen as less competent, and 3) fear of being seen as lazy. This may explain why only 5 of 37 enterprise pilots are getting to production on average and only 3 of these are creating real impact. So what is at the heart of this challenge?
For the less experienced workers, the challenge could be the lack of basic AI literacy or “tech savviness.” Only 40% of executives say their organizations have formal AI training for employees, according to Pluralsight. If you asked most workers to use AI to make their work more efficient, they just don’t know what to do. To compound this, most companies have no clear rewards or incentives for an employee who discovers a way to do their job faster and cheaper. So why would they raise their hand and share this with their manager when the downside is they could end up having to sign up to doing more of the same with fewer hours, or worse, potentially getting downsized?
If employee behavior change is at the core of AI transformation, then we first need to understand what drives behavior change in individuals and how we might adapt this in the context of AI.
For the subject matter experts (SMEs), the challenge is even more pronounced given SMEs play a key role in training/fine-tuning large language models (LLMs) for specific company use cases through human feedback and producing much of the unique content that these models operate on internally. Without them, the models are not only significantly less valuable and differentiated versus those used by other companies, but SMEs also provide the backstop for AI errors and are ultimately the ones needed to sign off and be accountable for specific AI performance in their domains.
Beyond these issues of skill level, employees bring varying psychological relationships with technology — what we call “Digital Temperament.” Those with higher Digital Temperament naturally approach new tools with curiosity and resilience, while those with lower Digital Temperament often experience genuine anxiety when faced with unfamiliar systems. Like other personality traits, Digital Temperament tends to remain stable over time, which helps explain why traditional training alone often fails to drive sustained adoption. Understanding this psychological dimension is crucial as we consider how to create lasting behavior change.
Creating Sustainable Behavior Change for AI Adoption
If employee behavior change is at the core of AI transformation, then we first need to understand what drives behavior change in individuals and how we might adapt this in the context of AI.
Employee AI adoption depends on a number of key factors including the individual’s personal characteristics (like temperament and having an open mindset) and environmental factors that can be improved through targeted interventions or “prompts” to promote sustained use. While personal characteristics are challenging to reliably change, it is possible to modify environmental factors to cause predictable behavior change. To explain this, we will use the Fogg Behavior Model adapted for AI adoption (shown in the figure below) as our guide. The Fogg Behavior Model states that three elements must come together at any point in time for a behavior to occur: 1) Motivation 2) Ability 3) a Prompt.
In order to move employees along the ability axis, we need to expose them to training, mentoring, and real-world experiences that can allow them to progress from awareness to proficiency to mastery of AI relative to their role. To move these same workers along the motivation axis, we first need to give them permission and ensure they are open-minded to AI experimentation, then provide a clear path to rewards and job satisfaction by using and creating benefits with AI, and then ultimately instill a sense of accountability and ownership of the AI solutions they participate in creating. Without progress on these two dimensions, it would be hard for most employees to think creatively about how to design the best AI prompt and what other information or inspiration they can give an LLM to accomplish specific tasks, let alone drive bigger AI innovations. The prompts to help accelerate this progress towards the sustained AI transformation zone may include executive-level sponsorship, objectives and key results linked to AI adoption, explicit funding for AI initiatives across the business, the allocation of time for AI experimentation, and universal AI training programs.
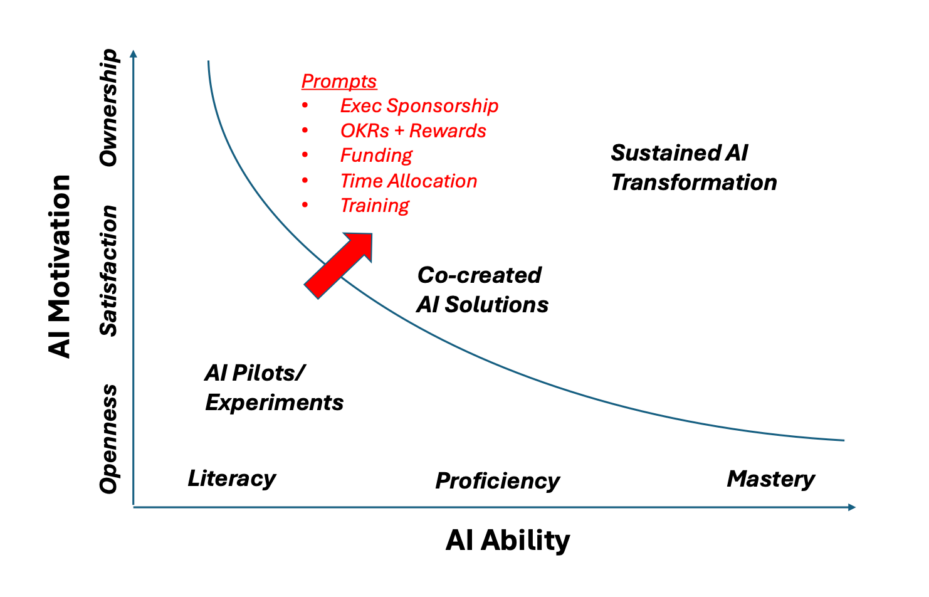
This adaption of the Fogg Model helps us understand why even motivated and trained employees might resist AI adoption. Beyond motivation and ability, the model highlights how “mental effort” and “social deviance” can create barriers. This explains why success requires both reducing the complexity of AI interactions and addressing social concerns about appearing “lazy” or “incompetent.” Organizations can address these barriers by designing more intuitive solutions while fostering a culture where leadership openly acknowledges their own AI learning curve and normalizes experimentation.
By establishing the ingredients for positive AI adoption and behavior change at the employee level, organizations can move beyond stalled AI pilots and experiments into co-created AI solutions and ultimately sustained AI transformation.
By establishing the ingredients for positive AI adoption and behavior change at the employee level, organizations can move beyond stalled AI pilots and experiments into co-created AI solutions and ultimately sustained AI transformation.
Clearing the Path to AI Adoption
To mobilize the organization on this path to transformation using the AI adoption model above, there are several foundational steps that will help clear the path.
1. View Time as Capital
Ultimately, giving employees the authority to use their “time as capital,” just as a VC would, provides a clear incentive to identify AI-driven efficiencies and time savings if these “saved hours” could be reallocated to more interesting tasks like innovation initiatives or additional AI training. For less experienced employees, this could include basic AI upskilling as well as refining creative and critical thinking skills to ensure they can navigate the “jagged frontier” of AI reliability for different use cases and judge AI outputs for potential ethics and safety risks. For experienced employees and SMEs, this could be focused training to work alongside AI to offload their menial tasks and allow them to review and sign off on high-impact AI solutions as well as redirect more of their time towards innovating high-risk/high-payoff AI use cases for the company. This could eventually form the basis for a new employee contract where workers gain more agency and ownership over how they co-create new AI solutions and share in the benefits with the organization. This reframing also helps reduce fear — employees no longer see efficiency gains as a threat to job security but rather as freed-up time they can reinvest in higher-value work.
2. Show People What Good Looks Like
Giving people examples of AI use cases related to their role and showing how these can be created will certainly “prime the pump” for those employees that are already past the “openness” phase and ready to explore driving real benefit with AI. But this may be less than 20% of the employee base in most companies. Most employees do not walk around dreaming of ways to apply AI to their everyday work or company challenges. Moving the other employees along will require personalized training but also peer mentoring along with permission to experiment (and fail) once they have a sense of what’s possible with AI. Without these additional elements, basic AI training and success cases may fail to drive real progress outside of the advanced group. Formal or informal “AI champions” within teams can share practical tips and lower the perceived stigma around using AI. This peer influence can be more powerful than top-down mandates.
3. Have Employees Rewrite Their AI-first Roles
To move towards the “ownership” level on the Motivation axis, employees have to not only engage in co-creating and being accountable for new AI solutions, but they also need to rethink their own role in the company and how this will change in an AI-first future. By allowing employees to help define/shape what their role could be in terms of activities, skills, experiences, and attributes, they will feel more empowered to work backward to identify what they need to start working on today to best prepare and create a positive path towards their future role (or other AI-first roles). Very few organizations have taken this key step to redefine the core roles in their company in an AI-first future, let alone have their employees participate in this. When employees participate in shaping AI’s place in their job, they’re more likely to see AI as an “enhancer” of their professional identity rather than a threat. This shift helps reduce resistance rooted in fears of being replaced.
4. Rethink the User Experience for AI Solutions
To maximize AI adoption, solutions should meet users where they are by:
- Embedding AI capabilities directly within existing workflows and tools
- Offering proactive suggestions instead of requiring user prompts
- Emphasizing curation over creation through pre-filled options and templates
- Using familiar interface patterns that feel natural to employees
This approach acknowledges that most users excel at selecting and refining AI-generated options rather than creating them from scratch. By reducing the mental effort required to engage with AI, organizations can lower adoption barriers for hesitant employees while making the technology feel like a natural extension of their work.
5. Create Peer Support Networks
Establish small “AI champion” teams or buddy systems where employees who have successfully integrated AI into their work can share practical tips with others. This approach not only reduces anxiety but also normalizes everyday usage of AI, especially for employees who may have lower Digital Temperament or are hesitant to try new tools.
How do we know when we are in the AI transformation zone? When we have elevated our employee base along the Motivation and Ability dimensions through thoughtful interventions or prompts, evolved our talent pool in terms of AI skills and mindset, and created AI solutions that lower the thresholds for engagement and impact for both employees and customers in the future. It’s also critical to measure real adoption — voluntary usage, time saved, and improved work quality — rather than relying solely on training completion or the number of pilot projects. By tracking these deeper indicators, organizations can see if AI has truly become a natural part of everyday work. These steps give us the opportunity to bring everyone along on our AI transformation journey so that both individuals and the organization overall can realize the benefits of the AI revolution.